Quality Assurance of Artificial Intelligence Systems
With increasing adoption of AI in software and cyber-physical systems, the system behavior is rapidly evolving from a rule driven response to intelligence driven response. Such a response is dynamic and data driven and is never deterministic thus rendering the conventional testing and monitoring paradigms ineffective. Moreover, since the system behavior is autonomous at run time, there are ethical, transparencies, regulatory and compliance issues that need to be validated, monitored and assured before the system is deemed fit for production
Six angles of quality for Artificial Intelligence
The illustration below depicts the six different angles that are used for digital assurance and testing of modern technology such as Artificial Intelligence, Robotics, Machine Intelligence and Cognitive IT. The first two angles (Mechanical and Electrical) only apply to physical robots and other smart devices/machines. Methods and techniques for assurance and testing of the mechanical and electrical aspects of machines have existed for a long time and are not particularly different for new technology. The third angle (Information Processing) relates to traditional IT-functions and systems. For this angle we have methods such as the TMap Suite available. The TMap suite is well-documented in books like “TMap NEXT”, “TMap HD” and “IoTMap”. And, of course, the website www.TMap.net gives a wealth of knowledge on testing. The new angles are quality assurance for Machine Intelligence and for the Business- and Social impact this new technology can have
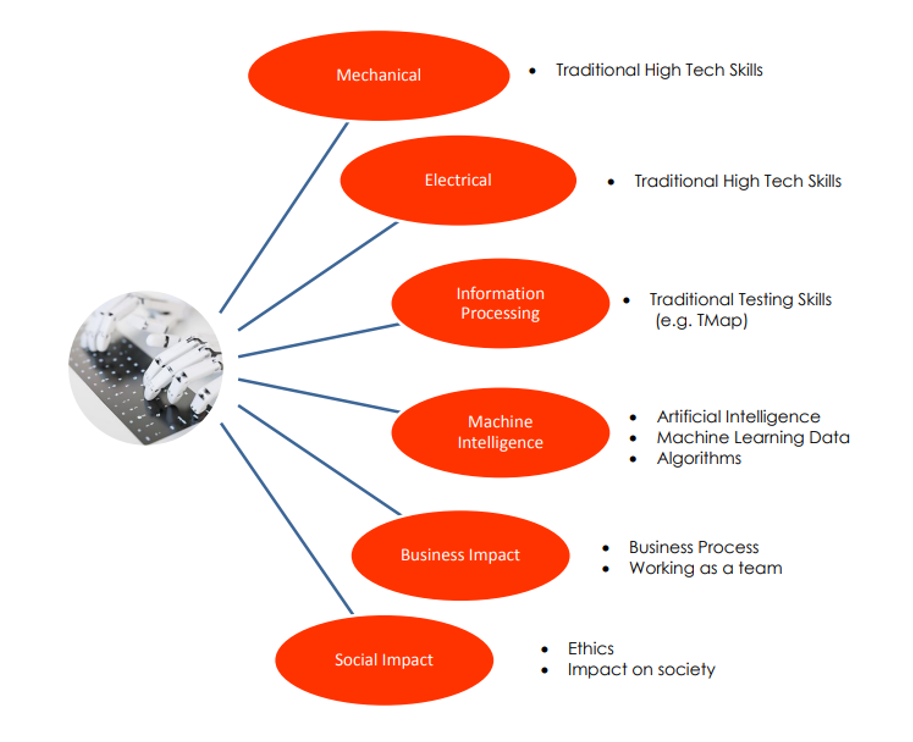
The Right Testing Strategy for AI Systems
At Infigrid, we define a comprehensive test strategy for AI system with specific testing techniques to mitigate risk of failure. Here are key AI components that must be tested to ensure proper AI system functioning.
Testing standalone cognitive features
Testing standalone cognitive features such as natural language processing (NLP), speech recognition, image recognition, and optical character recognition (OCR)
Data source and conditioning testing
Our AI Test Professional verifies quality of data from various systems – data correctness, completeness along with format checks and pattern analysis. Verify transformation rules and logic applied on raw data to get desire output format.
Algorithm testing
During this test phase, we verify input request and response from each API, verify request response pairs, test communication between components, response format and correctness, and conduct integration testing of API and algorithm.
System/regression testing
We execute end-to-end implementation testing such as provide an input, verify data ingestion, test the algorithm, communication through the API layer, and reconcile the final output on the data visualization platform with expected output.
Test Predictive Model
To test the predictive analytical model, we split the historical data into “test” and “train” datasets and train and test the model based on generated datasets. As a part of test result, we report the accuracy of model for the various generated scenarios.
Test AI-powered solutions
During this phase, we test AI-powered solutions such as virtual assistants and robotic process automation (RPA) such as test the chatbot framework, generate automated scripts in Python for execution, use open source automation tools such as Selenium, Sikuli and combinations of pattern, text, voice, image, and optical character recognition testing techniques with functional automation for true end-to-end testing of applications.
If you have any kind of AI testing requirement, feel free to get in touch with us or talk to one of our representatives and we will get back to you within 24 hours.
Our team is eager to start creating and get our hands and minds occupied with your next big thing. So, really, there's no reason to wait, get in touch and let's create together!